Food inspectors and public health officials generate a massive volume of inspection reports every year. Each of which needs to be reviewed and analyzed—a process that takes thousands of man hours. To Tom Sabo, Principal Solutions Architect at SAS, this is exactly the kind of problem that artificial intelligence/machine learning (AI/ML) and data analytics are poised to address.
He worked with more than 10 years of data from the Chicago Health Department, using visual text analytics to review and analyze 92,000 free-form statements within inspection reports to extract actionable data. In November 2023, Sabo presented his findings at the American Public Health Association (APHA) annual conference.
We spoke with Sabo to learn more about his findings, how these tools can be utilized by public health agencies and the food industry and what’s next for AI/ML in food safety.
Before we jump to your work with the Chicago Department of Health, can you explain what visual text analytics is and how you used it in this project?
Sabo: SAS has a platform called Viya 4.0, which is a platform for data analytics, data management and visualization. Visual text analytics is a set of capabilities within this platform that perform text analysis, which is a way to process documents—or the information within documents—to better understand themes, extract key information and look for particular patterns in an automated manner.
In the context of food safety inspections, two of the things I looked for in the inspection reports were very serious issues, such as mentions of pest violations, and where those violations occurred. Then I tied them together by asking the platform to show me, for example, all the cases where there was some kind of pest issue in relation to where that pest issue was discovered, because that is now actionable information. Text analytics is what allows us to identify those patterns.
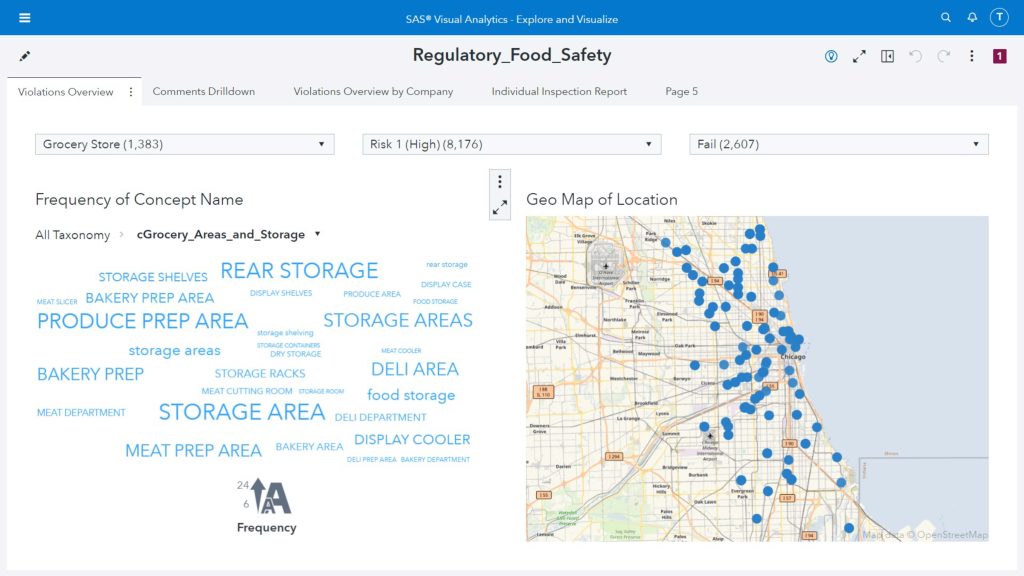
Can you tell me a little bit about the project you did with the Chicago Health Department?
Sabo: I didn’t work directly with them, but I did work with their data, which is publicly available. We pulled down their data from 2010 to 2021, which turned out to be 11,000 inspection reports done within the city of Chicago. Then we extracted out from the narratives freeform statements about specific issues and where they were identified.
This amounted to 92,000 freeform statements extracted from 11,000 reports. If someone were to manually go through those reports to identify the main issues mentioned, even if they spent only five minutes on a statement, it would amount to about 7,700 hours or four full-time employees working for one year.
We did talk to employees at public health agencies who said, if you are able to use these tools to help us better understand where our inspectors should spend their limited time, that would be very helpful to us. That’s why we started with serious issues. Our goal is to identify where the serious issues are occurring and answer questions about those incidents in the form of visual dashboards. And now we are adding generative AI, which helps us better communicate this information—what are the key issues and what can I do to prevent them—to folks who are not data scientists.
How does the platform report this information?
Sabo: We can generate a summary. For example, I would ask the large language model a question such as where should inspectors focus time and attention? And I would get a summary along the lines of “inspectors should focus their attention on areas where there is evidence of mice, rats, roaches and insects, including under prep tables, in produce areas, cabinets, all storage areas, basements and attics.”
Then, because the reports have geospatial information, I can drill down into the statements from the inspection reports that mention these areas of concern and stand up a map to see where issues occurred.
We could also do pretty fantastic things like look at an entire chain, such as all the 7-Elevens in the region, and assess across them where major issues were occurring. Inspection services could then get proactive and send that letters to these organizations stating, if you’re a convenience store, these are the areas that we recommend you do work in to prepare for your inspection: seal of the wall areas, ensure your door sweep is large enough to prevent rodents from coming into the establishment, etc.
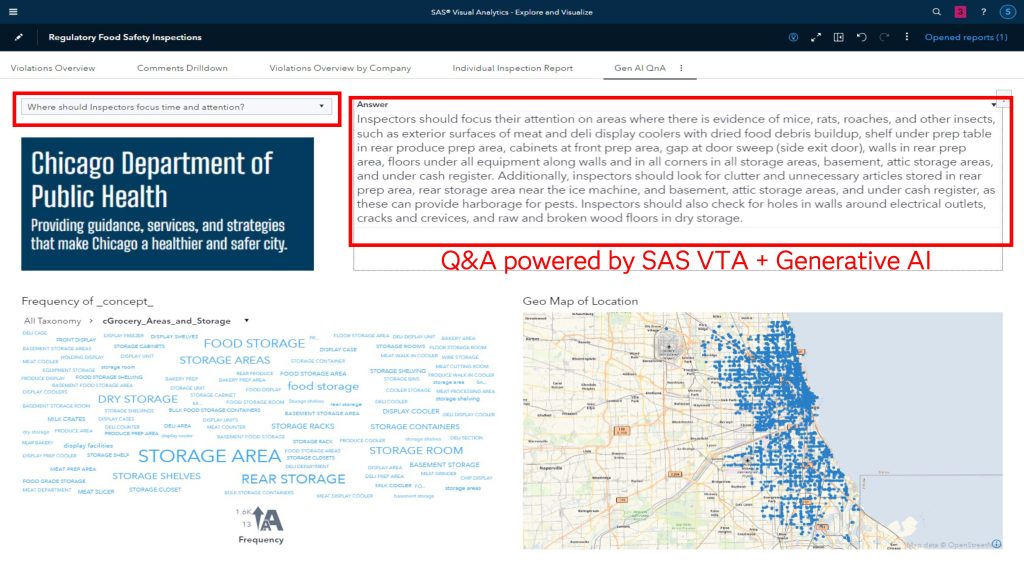
So you are reducing man hours in the review process and also providing more guidance to inspectors or establishments on where and what type of violations are occurring most frequently?
Sabo: Right. You can quantitatively determine that X out of 100 issues were related to storage areas, so when your inspectors come in, they can go back to the rear storage areas and make sure that clutter is removed and there is adequate lighting, for example. It helps inspectors focus in on the right places based on the evidence of where the issues actually occurred and were logged. And the algorithms we use can weight information based on what’s more recent. So maybe it would consider all the information it has been fed, but pay a lot more attention to the main issues in the last year.
What does the generative AI bring to the table that you don’t already have available with the text analysis?
Sabo: I come from a text analytics background. I’ve been doing this kind of work for years where I’ve been surfacing patterns and themes. What the generative AI (Gen AI) brings to the table, generally, is better communication of what’s going on at a high level. It allowed me to add a component to the dashboards that summarizes key questions, particularly around where should inspectors focus their time and attention.
For example, one of the questions I asked and could get an answer to from the Gen AI was, where are pest issues and where do they generally occur? I did not just go on my phone and hit ChatGPT. I fed the large language model with 1,500 statements—out of those 92,000 statements—that very specifically mentioned a pest issue in context of the location where it occurred. Once I fed my large language model with that data, I could then ask questions based on that data. I narrowed its domain so it could give me more focused answers that were more accurate. Based on this use case and many other use cases, I’ve seen the benefits of using text analytics as essentially a pre-filter for the large language models to focus in on key problems and answers.
Will the Gen AI tell you if it doesn’t have enough data to answer your question?
Sabo: It depends on how you work the large language model. In the case I’m working on, if I ask it something outside of its domain it will generally tell me, I don’t have enough information to answer that.
What is your next step in terms of developing or offering these tools?
Sabo: Now that we have done this work and proved it out with this set of data, the next step is to work with more agencies to help their overall public health strategies at the state and local level. We have worked with the FDA on using these capabilities to prevent chemicals from getting into the food supply. We have looked at drug and medical device safety and we’ve done work with the USDA FSIS looking at meat processing and using ML to help inspectors better prioritize their time based on the facilities that were most likely to have potential issues, based on past reports.
This platform is something that we could work with organizations on tomorrow. It took a matter of weeks to stand up the data that I presented at AHPA from the Chicago Health Department, so SAS is pretty well poised to work with any public health organization that’s interested in better understanding information from their inspection reports.